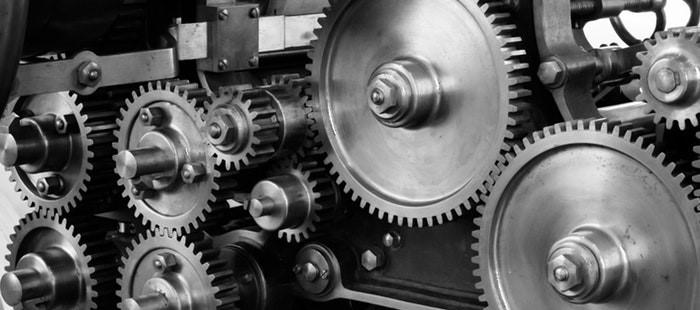
Predictive maintenance for factories is a method of preventing future machinery failure by analysing data during production to detect abnormalities ahead of time, so appropriate measures can be taken before damage can be done to the production line.
Up until recently, factory supervisors and machine operators would manually carry out scheduled maintenance in order to identify parts that may need to be repaired.
Why is Predictive Maintenance So Important?
In addition to using up valuable resource and productivity, it is thought that half of all preventative maintenance measures are actually ineffective. As such, it’s not surprising that predictive maintenance is quickly becoming a prominent Industry 4.0 technique for manufacturers.
Rolling out industrial IoT (Internet of things) technologies to monitor the condition of assets, optimise maintenance schedules, and gain real-time data, allows manufacturers to lower service costs, maximise output, and improve production rates.
The Difference Between Preventive and Predictive Maintenance
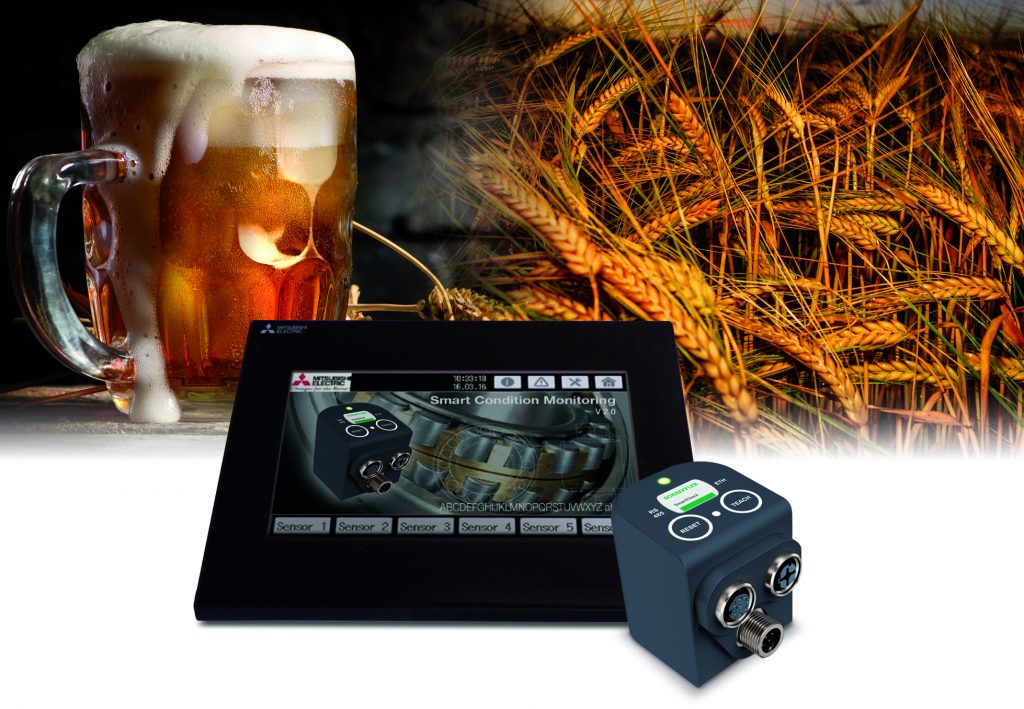
Manufacturers have been undertaking varying forms of preventive and predictive maintenance for many years. However, understanding the difference between the two, is critical. Preventive maintenance uses visual inspections, coupled with routine machinery monitoring. This, however, provides only limited, objective insight into the working state of a machine. During this process, engineers will regularly maintain and repair a machine to prevent possible failure. Predictive maintenance, however, utilises a data-driven approach, relying on analytics for maintenance and repair, to prevent issues ahead of time.
How Does Predictive Maintenance Work?
For predictive maintenance to be rolled out to an industrial asset, the following base components are needed:
- Sensors – installed to collect data in the product or machine
- Data Communication – allows the communication system to transport secure data between the asset and the data storage system
- Data Storage System – the central storage hub stores any asset and business data, and processes it either on-site or through the cloud
- Predictive Analytics – this form of analytics applies algorithms to the stored data to search for patterns and generatedashboard insights and alerts
- Root Cause Analysis – this form of data analysis used by maintenance and engineering specialists investigates insights and determines which actions should be performed.
Asset data from production is channelled from the sensors to a central repository using industrial communication gateways. Data from MES systems, along with manufacturing flow, are then integrated into a central data storage system to provide a further understanding of production asset data. Once predictive analytics are applied, it can then offer insights into how to reduce machine downtime, which will be investigated using root cause analysis software.
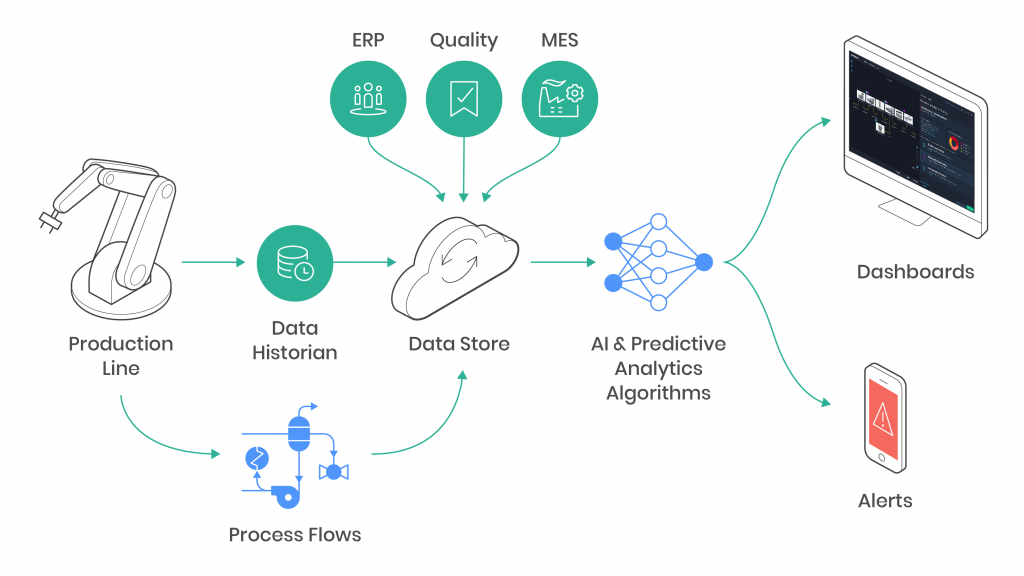
To roll out a predictive maintenance system properly, factories must first understand what failure is for each machine and create an interconnected system, featuring manufacturing assets, sensors, business systems, communication processes, cloud, predictive analytics and visualisation.
Using a visual modeller, engineering teams can capture the production line on the shop floor in a series of graphics. These include data flow, dashboards and ‘logic’ of the systems – where rules monitor and alert to any anomalies in the systems function to produce a system blueprint.
From here, predictive analytics are applied to machine data, and then the system blueprint, to predict the conditions which proceed a failure.
Benefits of Predictive Maintenance
By choosing to rely on predictive maintenance, businesses can benefit from two major advantages, such as:
- Reduction in Maintenance Time – automating reports for strategic maintenance and proactive repairs reduce maintenance time by 20-50% and decrease overall maintenance cost by 5-10%, according to Deloitte.
- Efficiency Increase – insights driven by analytical data improves the overall effectiveness of the equipment by reducing superfluous maintenance, extending the life of the machinery and, of course, uncovering any issues ahead of time.
Predictive Maintenance Equipment
Predictive maintenance equipment will require an IoT platform for industry which is used to model, simulate, test and deploy the predictive maintenance solution. Also, included are industry data integrations and analytic algorithms to pinpoint issues in machine data, and root cause analysis to investigate the insights to determine what action is to be taken.
How Can Factories Use Predictive Maintenance?
We’ve seen organisations implement predictive maintenance in a range of different ways, from factory-wide deployment to more targeted solutions for a single machine part.
For factories producing parts and machinery, a common measure is to monitor and inspect the condition of a motor to receive alerts based on its productivity, power consumption, health status, and internal wear and tear.
Another practical use of predictive maintenance is minimising product defects and waste reduction. This is often known as Quality 4.0, and implementations such as these, can predict when the number of defective products will exceed the threshold percentage, and what the causes are for the failure.
Factories are also increasingly turning to predictive maintenance for Factory 4.0. This creates a connected factory, with the use of sensors in machines, workstations and other important sites across the factory to predict issues.
Common Predictive Maintenance IoT Approaches
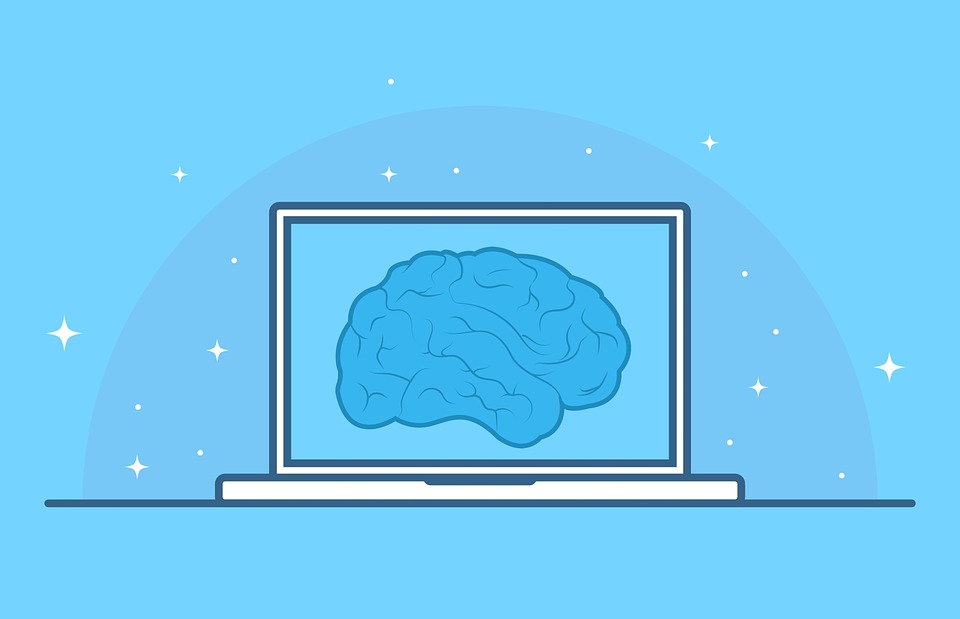
The two most common approaches to predictive maintenance are machine learning-based and ruled-based.
Machine Learning-Based
Industrial AI can be applied to predictive maintenance and many other facets of the manufacturing process. Although we are only just beginning to understand and deploy this technology, many factories across the UK and abroad are benefiting from machine learning.
AI is ideally placed to work alongside predictive maintenance. It offers a user a whole host of techniques to understand and analyse considerable chunks of data collected during the manufacturing process to deliver actionable insights to sustain high-manufacturing levels. These techniques are more commonly referred to as Machine Learning Algorithms.
What Are Predictive Maintenance Algorithms?
Advanced AI algorithms are used to learn how a machine typically behaves in order to use this as a baseline to identify real-time deviations in performance. The algorithms used for machine learning, as we’ve already touched upon, must analyse input historical or test data and output data. A machine monitoring system includes input on a selection of different factors such as temperature, pressure and motor and engine speeds.
Using machine learning in predictive maintenance involves combining machine-learning algorithms with large subsets of, either historic or test data, in varying scenarios to understand and predict, why, when, and how something can go wrong.
There are two main kinds of AI and machine learning used in predictive analytics – supervised and unsupervised. Both of which are relevant depending on the circumstances, and the availability of test and historic data.
Rule-Based Predictive Maintenance
Also, known as condition monitoring, rule-based predictive maintenance relies on sensors which are always collecting data about assets; sending alerts according to predefined protocols, when a specific rule has been reached.
Rule-based maintenance requires production teams to work closely with engineering and customer-focused teams to establish the causes and contributing factors that may lead to machine failure.
Once these factors are established, manufacturers can produce a virtual model of their connected system. Here they can map out the behaviours and inter-dependencies between the various IoT components.
For instance, if temperature and rotation speeds go above or below predefined readings, the system will then alert the dashboard, which will then be read by an engineer who can address the issues before complete failure occurs.
Although these rules provide some level of automated, predictive maintenance, they are still somewhat dependent on the team’s understanding of what components or environmental circumstances need to be measured.
If you require any further information about anything we’ve covered in this piece, or you’re interested in any of our other solutions, then why not contact us today?